Data quality is a critical aspect of any organization’s data management strategy. Poor data quality can lead to numerous issues, including inaccurate reporting, flawed analysis, and inefficient operations. One effective solution to address data quality issues is to implement a fully managed data matching solution.
In this quick post, we will explore the five compelling reasons why businesses should consider adopting a fully managed data matching solution to overcome data quality challenges. By understanding the data matching benefits, organizations can improve data accuracy, enhance decision-making processes, and achieve better overall data integrity.
What Does Fully Managed Data Matching Mean?
A fully managed data matching solution refers to a comprehensive approach where an external service provider takes care of all aspects of the data matching process on behalf of an organization. In this setup, the service provider handles the entire lifecycle of data matching, from data preparation and cleansing to executing the matching algorithms and delivering the matched results.
With a fully managed data matching solution, organizations can offload the complexities and resource-intensive tasks associated with data matching to a specialized team. The service provider typically offers a cloud-based platform or software-as-a-service (SaaS) solution that enables seamless integration with the organization’s existing data systems.
The service provider takes responsibility for ensuring the accuracy and efficiency of the data matching process. They employ advanced matching algorithms, such as fuzzy matching, phonetic matching, or statistical techniques, to identify and link similar or related data records. The solution is designed to handle diverse data sources, formats, and complex matching requirements.
A fully managed data matching solution often includes data cleansing capabilities to address data quality issues. This may involve standardizing formats, correcting errors, removing duplicates, and validating data against predefined rules or reference datasets.
By opting for a fully managed data matching solution, organizations can benefit from the expertise and experience of the service provider. This eliminates the need to invest in developing in-house data matching capabilities, maintaining infrastructure, and training staff. It allows organizations to focus on their core operations while ensuring that data quality issues are effectively addressed.
Overall, a fully managed data matching solution offers a comprehensive and hassle-free approach to data matching. It streamlines the process, improves data accuracy, and provides organizations with reliable and high-quality data for their decision-making processes.
Why Do Database Administrators or Analysts Need Fully-Managed?
Database administrators play a crucial role in managing and maintaining the integrity of an organization’s databases. When it comes to data quality issues, such as duplicate data and inaccurate data, manual data matching can present significant challenges. This is where a fully managed data matching solution becomes essential for database administrators. Here are some reasons why they need a fully managed data matching approach:
- Efficiency and Time Savings: Manual data matching requires significant time and effort from database administrators. They need to manually compare and match records, which can be a tedious and error-prone process. A fully managed data matching solution automates the matching process, reducing the time and effort required. This allows database administrators to focus on more strategic tasks rather than spending valuable time on repetitive data matching tasks.
- Accuracy and Data Integrity: Manual data matching is prone to human errors, especially when dealing with large datasets. Inaccurate or incomplete data matching can lead to duplicate data in the database, affecting data integrity and causing inconsistencies in reporting and analysis. A fully managed data matching solution utilizes advanced matching algorithms and techniques, ensuring accurate and reliable results. This helps maintain data integrity and improves overall data quality within the database.
- Scalability and Handling Complex Matching Requirements: As databases grow in size and complexity, manual data matching becomes increasingly challenging. Database administrators may struggle to handle the volume of data and the various matching scenarios that arise. A fully managed data matching solution is designed to handle large datasets and complex matching requirements. It offers scalability and flexibility to adapt to changing business needs, ensuring efficient data matching even as the database expands.
- Consistent Data Governance and Compliance: Data governance and compliance are crucial aspects of database management. Manual data matching may lack consistency in applying data governance policies and compliance regulations across all matched records. A fully managed data matching solution can integrate with data governance frameworks and enforce standardized data practices. This ensures that data matching processes adhere to compliance regulations and organizational policies consistently.
- Advanced Data Cleansing and Deduplication: Duplicate data is a common issue that affects data quality and database performance. Manual data matching may not effectively identify and remove duplicate records, leading to data redundancy and inefficiency. A fully managed data matching solution incorporates data cleansing capabilities, including deduplication techniques. It can identify and merge duplicate records, improving data quality and optimizing database performance.
Summary
In summary, a fully managed data matching solution is crucial for database administrators to overcome the challenges associated with manual data matching. It provides efficiency, accuracy, scalability, compliance, and advanced data cleansing capabilities, enabling administrators to maintain data quality, integrity, and reliability within their databases. By adopting a fully managed approach, database administrators can focus on strategic tasks and ensure the effective management of data quality issues.
Continue Reading:
Real-Time ETL vs Batch ETL: Types of Data Integration
What is Data Science? Life Cycle, Applications & Tools
ABOUT THE AUTHOR
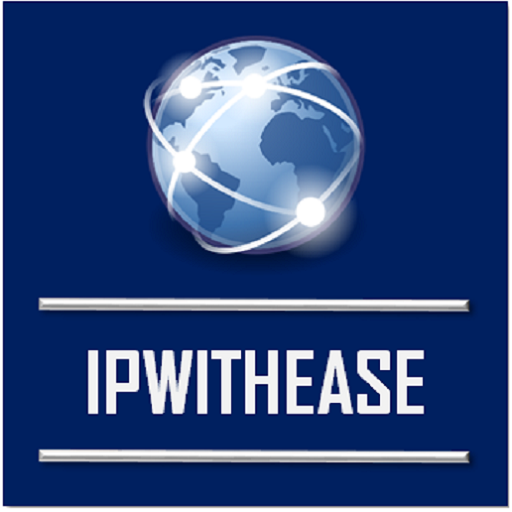