Table of Contents
In the rapidly evolving landscape of education, artificial intelligence (AI) is transforming how personalized learning is delivered. At the forefront of this shift is RAG AI (Retrieval Augmented Generation AI), a technology that integrates large language models (LLMs) with dynamic information retrieval to create tailored and adaptive educational experiences.
RAG AI is reshaping the future of education by offering real-time personalization that addresses the unique needs of individual learners.
The Promise of AI in Education
AI has long been seen as a solution to the challenges of delivering personalized learning experiences at scale. Traditional education systems often fail to accommodate the diverse learning styles, paces, and needs of students. RAG AI, by enabling AI-driven adaptive learning, provides a way to customize educational tools that respond dynamically to each student’s requirements in real time, improving both learning outcomes and engagement.
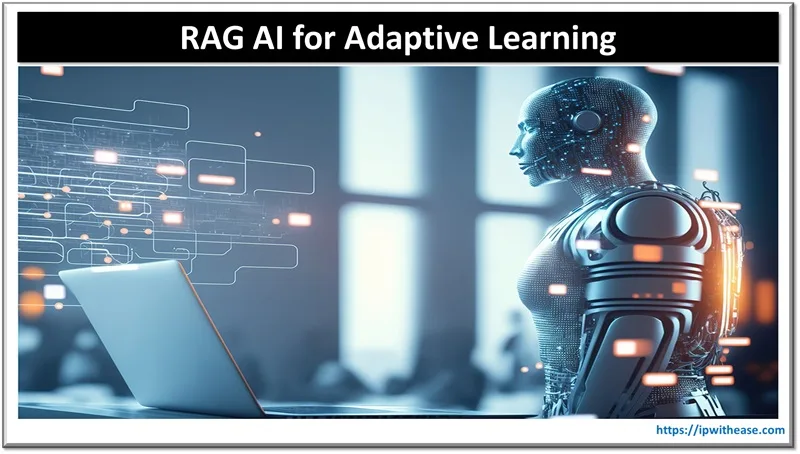
Understanding RAG AI in the Educational Context
RAG AI enhances the power of large language models by integrating real-time access to external knowledge bases. In education, this means that AI systems can pull from up-to-date educational content, student performance data, and pedagogical strategies, tailoring the generated responses and content to suit individual learners’ profiles.
Related: Generative AI vs ChatGPT
Key Components of RAG AI in Education
- Knowledge Base: A repository that houses educational materials, such as textbooks, lecture notes, and supplementary resources.
- Student Profile Database: A dynamic storage of individual student data, tracking progress, strengths, weaknesses, and learning preferences.
- Retrieval System: The intelligent mechanism that selects relevant information from the knowledge base based on student queries and profiles.
- Language Model: The AI capable of generating human-like text and interpreting complex educational queries to provide contextually appropriate responses.
Implementing RAG AI for Adaptive Learning
Building adaptive learning tools using RAG AI involves several critical steps:
1. Content Ingestion and Indexing
Educational content is processed and indexed to form a searchable knowledge base, including textbooks, articles, video transcripts, and other learning resources.
2. Student Profile Creation
AI systems collect and analyze individual student data, tracking their progress, quiz results, assignment performances, and interactions with learning materials.
3. Query Processing
When a student asks a question or seeks help, the RAG AI system processes the query in the context of the student’s unique learning profile and objectives.
4. Relevant Information Retrieval
RAG AI retrieves the most pertinent information from the knowledge base, factoring in the student’s current level of understanding and their learning trajectory.
5. Personalized Response Generation
Using both the retrieved information and the student’s profile, the AI generates a tailored response that addresses the student’s specific needs in an accessible and engaging manner.
Related: What is ChatGPT And How Can You Use It?
Benefits of RAG AI-Powered Adaptive Learning Tools
1. Highly Personalized Learning Experiences
RAG AI enables educational tools to provide explanations and examples that are tailored to each student’s background, learning style, and current knowledge level.
2. Real-Time Adaptation
As students make progress, RAG AI systems can adjust the difficulty and complexity of content to match their evolving capabilities, ensuring continued engagement and challenge.
3. Comprehensive and Up-to-Date Information
By accessing a vast and current knowledge base, RAG AI ensures that students receive the most relevant and accurate information, reducing the reliance on outdated materials.
4. Enhanced Engagement
RAG AI provides a personalized learning experience that boosts student engagement by presenting material in ways that are most appealing and effective for individual learners.
5. Efficient Knowledge Gaps Identification
RAG AI systems can quickly identify gaps in a student’s understanding and provide targeted support, ensuring that learning is both comprehensive and continuous.
Challenges and Considerations
While RAG AI holds significant promise for adaptive learning, several challenges remain:
- Data Privacy: Protecting student data and ensuring its ethical use is paramount.
- Content Quality: Maintaining a high-quality, up-to-date knowledge base requires ongoing curation and verification.
- Algorithmic Bias: Addressing biases within the AI system is crucial to ensure fair treatment of all students.
- Teacher Integration: Successful implementation requires seamless integration with traditional teaching methods and active involvement from educators.
The Future of RAG AI in Education
As RAG AI technology evolves, the future holds even more advanced adaptive learning tools, including:
- Multimodal Learning: AI systems that incorporate visual, auditory, and interactive elements to offer a more comprehensive and engaging learning experience.
- Collaborative Learning: Tools that facilitate group learning activities by intelligently supporting collaborative problem-solving and discussions.
- Predictive Analytics: Algorithms that forecast student performance and provide early interventions based on data-driven insights, helping students stay on track.
Unlocking the Future of Education with RAG AI
The integration of RAG AI in education is revolutionizing how personalized learning is delivered, making it more adaptive, responsive, and effective. By harnessing AI’s ability to retrieve up-to-date information and generate tailored content, RAG AI opens the door to truly customized learning experiences that can adapt in real time to the needs of individual students. This shift promises not only to make education more accessible and engaging but also to enhance the overall learning experience in ways that were previously unimaginable.
ABOUT THE AUTHOR
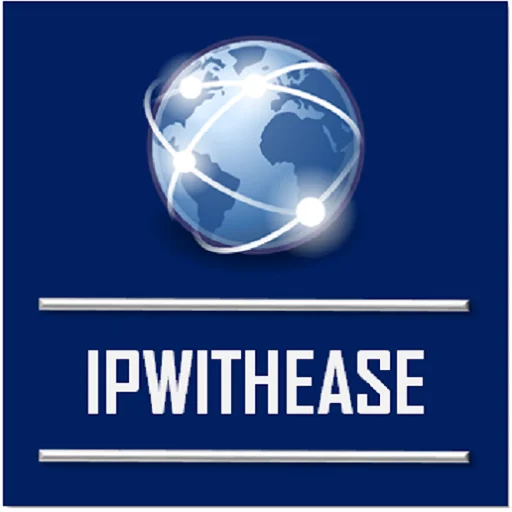
IPwithease is aimed at sharing knowledge across varied domains like Network, Security, Virtualization, Software, Wireless, etc.