Table of Contents
DDoS attacks are increasing, with a nine-year 807% increase in attacks and a total of 13 million in 2022. Those numbers will continue to rise year-on-year. But the issue is that DDoS attacks are so tricky to detect. Why? Read on to find out.
The Nature of DDoS Attacks
Basically, DDoS attacks involve overwhelming a target system, like a website or an online service, with massive amounts of internet traffic. Yes, you can prevent DDoS attack to an extent, but they’re so tricky to detect that you don’t know when they’re coming. The flood of internet traffic it creates is generated by compromised device networks called botnets.
DDoS attacks are so hard to detect because they can replicate legitimate traffic. Compared to other cyberattacks, which usually exploit vulnerabilities or involve malware, DDoS assaults involve flooding the target with an almost endless number of ordinary requests. The average DDoS attack uses 5.17 gigabytes per second of data, with some massive DDoS attacks surpassing 71 million requests on a network per second.
During peak usage time with naturally high traffic levels, standard security systems can’t decipher between genuine and malicious user activities.
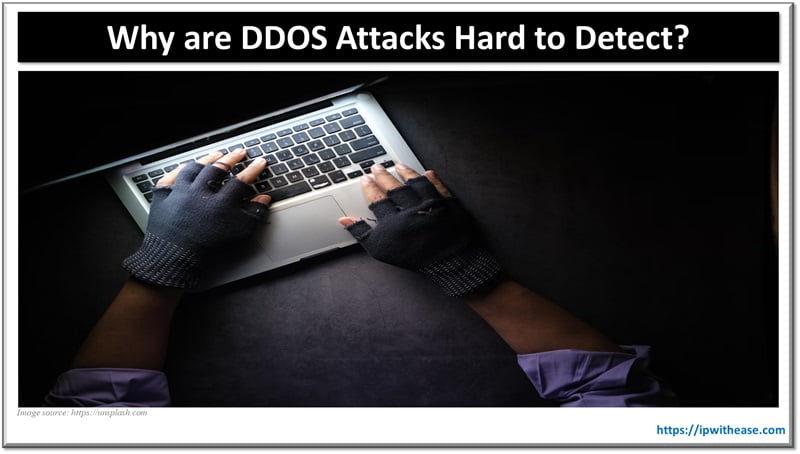
The Botnets
Botnets are essential in executing a successful DDoS attack since they offer the scale and spread necessary for effective operations. Each botnet consists of several thousands or millions of infected devices contributing insignificant quantities of attacking requests at the target. The distributed nature means multiple sources of this malicious traffic often exist across different geographical locations.
This distribution makes detection difficult. Traditional security measures – including firewalls and intrusion detection systems – might be able to identify and block traffic originating from a single malicious IP address. But when an attack originates from numerous IP addresses, these countermeasures lose their effectiveness.
Besides, blacklisting offending IPs becomes harder without unintentionally blocking genuine users because botnets use authentic devices that are already on blacklists.
Continual Change of Attack Techniques
Complicating detection efforts further is the fact that the methods involved in DDoS attacks are ever-changing. To avoid detection and maximize impact, attackers use different means. For example, they may engage in various DDoS attacks, like volumetric attacks, where a massive amount of traffic is directed at the target, or application layer attacks, designed for specific applications or services with few pointed requests.
Encryption is another technique that can be used to hide malicious traffic. Also, there may be amplification techniques including small requests producing big responses, leading to a significant increase in the amount of traffic sent to the target.
Basically, it’s constantly changing and tricky to follow.
Problems with Traffic Analysis
Detection of DDoS attacks depends on network traffic analysis through pattern and anomaly identification. However, this analysis presents specific challenges. First and foremost, it’s tricky to constantly monitor and analyze all the real-time traffic generated by huge networks due to their massive size. In a situation where an attack occurs, this situation gets even worse as the volume of data might multiply multiple times within seconds.
Attackers often mix malicious traffic with legitimate ones using sophisticated techniques. For example, this could involve launching their attacks during peak usage periods or masking sources using IP spoofing. Not only does this make it difficult for security systems to distinguish an alert from normal network activity noise, but it also causes a serious interruption in network activities, especially when they produce a lot of traffic, as indicated earlier.
The Need For Advanced Detection Strategies
Traditional security measures can’t tackle the complexities involved in identifying DDoS attacks. Organizations must opt for advanced detection techniques that exploit machine learning and artificial intelligence (AI) methods in real-time network traffic analysis and anomaly identification.
Machine learning algorithms can identify expected patterns of normal network behavior while at the same time detecting deviations that may be indicative of a DDoS attack. These systems can adapt as new attack methods emerge by using recent data to improve their detection abilities. And – AI-driven mechanisms respond automatically to incidents with measures like traffic redirection or deploying countermeasures, reducing the impact.
Naturally, sharing information and working together helps strengthen the DDoS detection system. That involves exchanging informed data about threats and attacks, enhancing comprehension of the basis on which defenses are built against DDoS attacks. Collaboration is probably one of the most effective strategies, but it isn’t always easy.
By the sounds of it, nothing about detecting DDoS attacks is easy.
DDoS attacks are one of the trickiest to detect and the most damaging. Downtime for a website costs the average business $427 each minute. Service providers must be proactive as these highly sophisticated strikes against websites. But as you can see from the information we’ve given you above, it’s not exactly simple to detect a DDoS attack, and it’s also not always easy to do something about them.
Continue Reading
DOS vs DDOS: Detailed Comparison
ABOUT THE AUTHOR
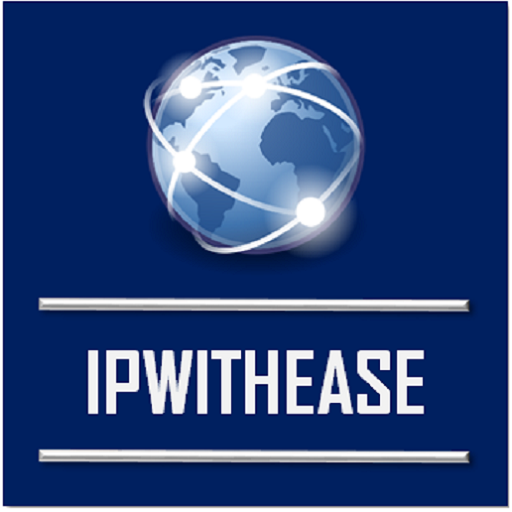
IPwithease is aimed at sharing knowledge across varied domains like Network, Security, Virtualization, Software, Wireless, etc.