Table of Contents
Generative AI, including models like ChatGPT, represents a significant advancement in artificial intelligence. These models are designed to generate human-like text based on the input they receive. Here’s a breakdown of what generative AI is and how ChatGPT fits into this category:
What is Generative AI?
Generative AI refers to a subset of artificial intelligence that focuses on creating content. This can include text, images, music, and more. The key characteristic of generative AI is its ability to produce new data that mimics the style and structure of the training data it was exposed to.
Applications of Generative AI
- Text Generation: Producing articles, stories, and responses to questions.
- Image Generation: Creating artwork or realistic photos.
- Music Generation: Composing original music pieces.
- Code Generation: Writing programming code based on descriptions.
How Does Generative AI Work?
Generative AI models are typically based on deep learning techniques, particularly neural networks. They are trained on vast datasets to recognize patterns and generate content that is coherent and contextually relevant. Key components include:
- Neural Networks: These are computational models inspired by the human brain, consisting of layers of interconnected nodes (neurons).
- Training Data: Large and diverse datasets are used to train models, helping them learn the nuances of language, image, or other content types.
- Training Process: During training, the model adjusts its parameters to minimize errors in predictions, learning to generate outputs that closely resemble the training data.
ChatGPT: A Case Study in Generative AI
ChatGPT, developed by OpenAI, is an example of a generative AI model designed for text generation. It uses a transformer architecture, specifically the GPT (Generative Pre-trained Transformer) model, to understand and generate human-like text. Here’s how it works:
- Pre-training: ChatGPT is pre-trained on a large corpus of text from the internet, allowing it to learn grammar, facts, and some reasoning abilities.
- Fine-tuning: The model is fine-tuned with additional datasets and sometimes with human feedback to improve its performance and make it more suitable for specific applications.
- Generation: When given a prompt, ChatGPT generates text by predicting the next word in a sequence, continuing this process until it produces a complete and coherent response.
Applications of ChatGPT
ChatGPT and similar models have a wide range of applications, including but not limited to:
- Customer Support: Automating responses to common customer queries.
- Content Creation: Assisting in writing articles, blogs, and social media posts.
- Education: Providing tutoring and answering educational questions.
- Entertainment: Creating interactive storytelling experiences and game dialogues.
Challenges and Ethical Considerations
While generative AI holds great promise, it also presents challenges and ethical considerations:
- Bias and Fairness: Models can inherit biases present in the training data, leading to biased or unfair outputs.
- Misinformation: The ability to generate realistic text can be misused to spread misinformation.
- Privacy: Ensuring that the data used for training does not violate privacy is crucial.
- Control and Safety: It’s important to develop mechanisms to control and ensure the safe deployment of generative AI systems.
Comparison: Generative AI vs ChatGPT
Below table highlights the differences and similarities between ChatGPT and generative AI:
Feature/Aspect | ChatGPT | Generative AI (General) |
Definition | A specific implementation of generative AI focused on text-based interactions. | A broad category of AI that creates content, including text, images, music, etc. |
Primary Function | Text generation, conversation, and answering questions. | Content generation across various media types (text, images, audio, video). |
Underlying Technology | Based on the GPT (Generative Pre-trained Transformer) architecture. | Various architectures, including GANs (Generative Adversarial Networks), VAEs (Variational Autoencoders), and transformers. |
Training Data | Large corpus of text data from the internet. | Depends on the application: text, images, audio datasets, etc. |
Applications | Chatbots, virtual assistants, content creation, education, and entertainment. | Diverse applications including image synthesis, music composition, video creation, and more. |
Output Type | Textual content. | Text, images, music, video, 3D models, and other forms of media. |
Interactivity | High interactivity, designed for real-time conversation. | Varies by application; can be interactive (like text-based chat) or static (like image generation). |
Examples | ChatGPT by OpenAI, GPT-3, GPT-4. | DALL-E (images), DeepArt (artwork), Jukedeck (music), StyleGAN (images). |
Ethical Considerations | Bias in responses, misinformation, privacy issues in training data. | Bias in generated content, deepfakes, intellectual property concerns, privacy issues. |
Strengths | Coherent and contextually relevant text generation, adaptable to various domains. | Versatility across different content types, ability to innovate in creative fields. |
Challenges | Maintaining relevance and accuracy, handling ambiguous or sensitive topics. | Ensuring realism and quality across different media, preventing misuse. |
Customization | Can be fine-tuned for specific tasks or industries. | Customizable based on the type of content to be generated and the specific application. |
Final Words
Generative AI, exemplified by ChatGPT, represents a transformative technology with the potential to significantly impact various fields. Ongoing research and development are crucial to harness its benefits while addressing the associated challenges. The comparison highlights how ChatGPT, as a specialized form of generative AI focused on text, fits within the broader landscape of generative AI technologies.
ABOUT THE AUTHOR
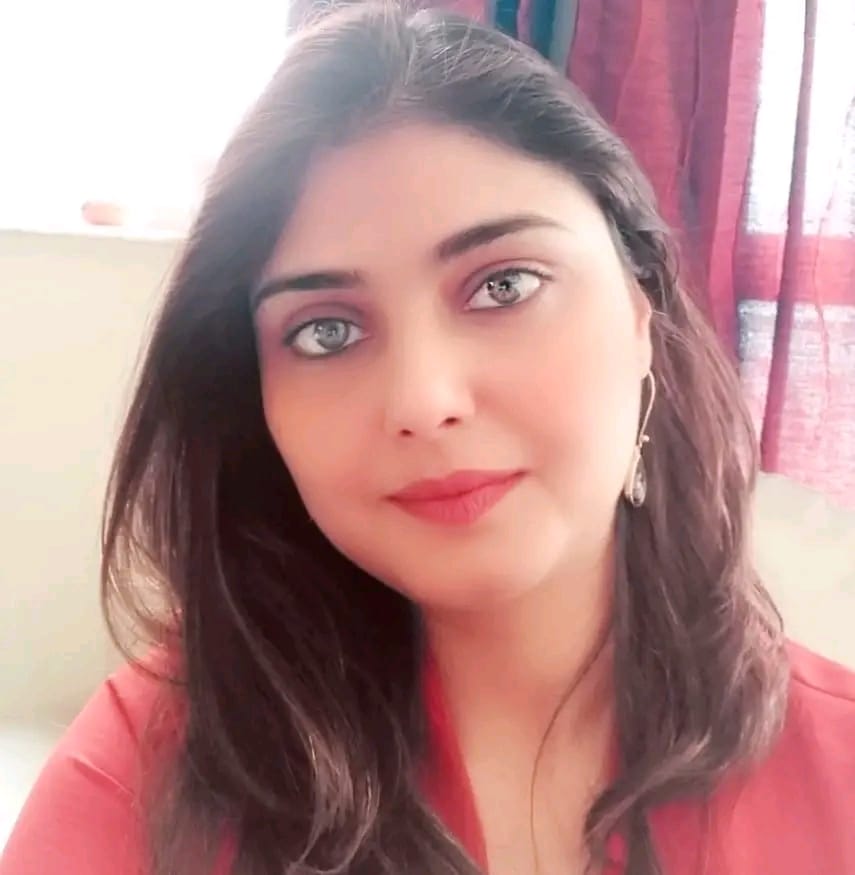
You can learn more about her on her linkedin profile – Rashmi Bhardwaj