Table of Contents
Introduction
Machine learning techniques have developed into useful tools for enhancing cybersecurity measures in response to the growing hazards and flaws in our digital world. These advanced systems are designed to sift through enormous amounts of data, quickly identifying unusual patterns that could signal a potential attack. By acting faster than older security methods, machine learning has the potential to outpace hackers and minimize damage. However, these tools aren’t without their challenges, which can sometimes limit their effectiveness.
How Machine Learning Is Used in Cybersecurity
Machine learning in cybersecurity is widely used to detect and prevent cyber threats by helping detect and stop threats faster and more accurately. ML tools analyze vast amounts of data to find out the patterns and unusual behavior, which can turn out to be an actual trend. For example, ML can detect unusual network activities, identify harmful software according to how it behaves, and block fake emails trying to steal information. It also helps predict future attacks by studying past data, allowing companies to stay ahead of hackers.
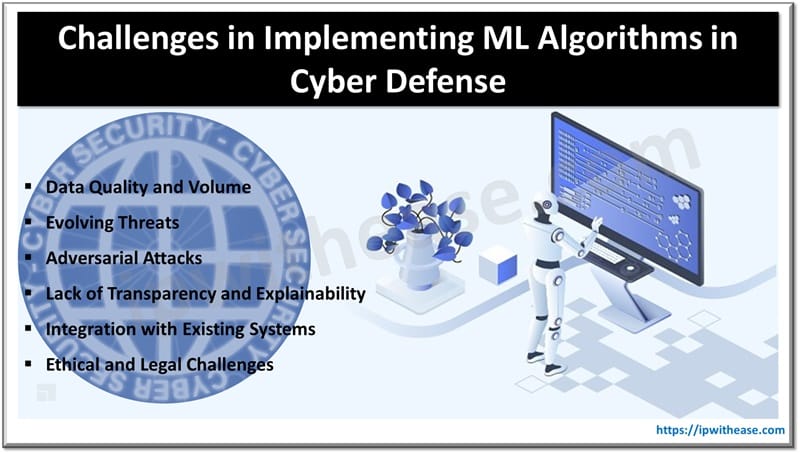
Challenges in Implementing ML Algorithms in Cybersecurity
Data Quality and Volume
The smooth operation of machine learning in cybersecurity requires high-quality data to learn from. Every day, businesses generate massive amounts of information, like logs, network traffic, and user activity. While this data is valuable, it can also be messy or incomplete, which might cause ML systems to make mistakes. For example, if a system is trained on data filled with false positives, it might wrongly flag safe activities as threats.
A lot of cybersecurity tools today struggle with this problem. Studies reveal that many alerts generated by these tools are false alarms, leading to wasted time and resources. To tackle this, companies must provide carefully curated datasets that help ML models learn to differentiate real threats from harmless actions, improving their accuracy. Without this, the efficiency of ML algorithms in identifying real threats may diminish significantly.
Evolving Threats
Cybercriminals are always changing their strategies, methods, and approaches to get beyond established defenses. While ML models excel at recognizing known attack methods, they may struggle to detect brand-new types of attacks, often called “zero-day threats”. This is a result of machine learning’s heavy reliance on historical data for the prediction, which is why the system might miss a new attack.
Modern cyberattacks, particularly ransomware, are becoming more sophisticated each year. In 2024, ransomware attacks have surged, with tactics like double extortion where attackers not only encrypt data but also exfiltrate it, using the threat of exposure as leverage, becoming more common. According to a report from the World Economic Forum, an increased utilization of AI by attackers is expected. They already use AI-powered tools like Generative AI, Voice simulation software, and deepfake video technology, which necessitates even stronger AI cybersecurity measures.
Adversarial Attacks
A particularly concerning challenge in implementing ML for cyber defense is the rise of adversarial attacks. The goal of these assaults is to take advantage of the flaws in machine learning models. Cybercriminals can modify the data supplied to an ML system, tricking the algorithm into classifying things incorrectly. For instance, attackers can elude detection by even the most advanced models by discreetly changing the properties of malicious files or traffic patterns.
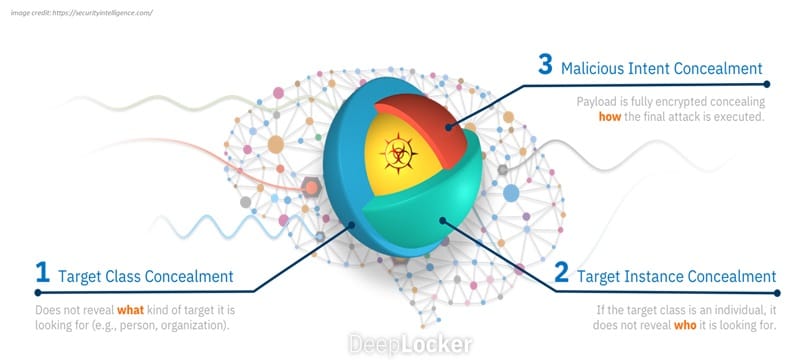
A real-world case of this was the “DeepLocker” malware, which used AI to hide malicious intent until a specific set of conditions were met. According to IBM Security Intelligence, DeepLocker, is a new breed of highly targeted and evasive attack tools powered by AI. DeepLocker hides its malicious payload in benign carrier applications, such as video conference software, to avoid detection by most antivirus and malware scanners. Such attacks demonstrate how adversaries can target the ML model rather than the network. Defending against these attacks requires continuous model retraining and the development of robust adversarial defenses – both of which are resource-intensive.
Lack of Transparency and Explainability
One of the biggest challenges in using ML for cyber defense is the black-box nature of many machine learning models. Algorithms can identify suspicious conduct, but they frequently don’t explain why a particular action was judged dangerous. Because of this lack of transparency, cybersecurity teams may find it challenging to accept the model’s conclusions or implement corrective measures.
An ML-powered tool, for instance, can notify a cybersecurity analyst that a specific IP address is linked to a possible assault. Nevertheless, it becomes challenging to verify the threat or do additional research in the absence of explicit logic or an explanation of how the algorithm arrived at that conclusion. This is the area where explainable AI, which seeks to create ML models, is becoming more popular.
Integration with Existing Systems
Most businesses have complicated cybersecurity architectures consisting of firewalls, endpoint security products, and legacy systems. Several obstacles prevent integrating ML algorithms into these current systems. Machine learning technologies frequently need a current infrastructure to process and analyze data effectively. The requirements of real-time data processing may be too much for older systems to handle, which lowers the ML model’s overall effectiveness.
Moreover, to integrate ML in cybersecurity, there’s a need for knowledgeable staff that have enough qualifications in cyber protection and machine learning.
Ethical and Legal Challenges
The implementation of machine learning in cyber defense also raises ethical and legal concerns. For example, for machine learning models to work properly, they frequently need access to private or business data. This calls into question issues with data security, privacy, and adherence to laws like the GDPR.
Companies need to find a way to use data security features without going overboard and still comply with all applicable data protection regulations. The way automated systems make judgments also raises ethical questions, particularly when obstructing acts by lawful users could hurt how businesses operate.
Conclusion
There is no doubt that machine learning is extremely useful in cybersecurity as it can analyze vast databases, swiftly react to attacks, and identify irregularities. But for machine learning to be completely effective in cyber protection, issues including data quality, changing threats, adversarial assaults, and integration barriers need to be resolved.
In the future, businesses should concentrate on employing carefully selected datasets, retraining models continuously, and developing transparent, intelligible AI systems that can seamlessly connect with current infrastructures. The instruments we employ to counter threats must also change as they do. These obstacles can be overcome and machine learning (ML) elevated to a central role in contemporary cyber protection.
ABOUT THE AUTHOR
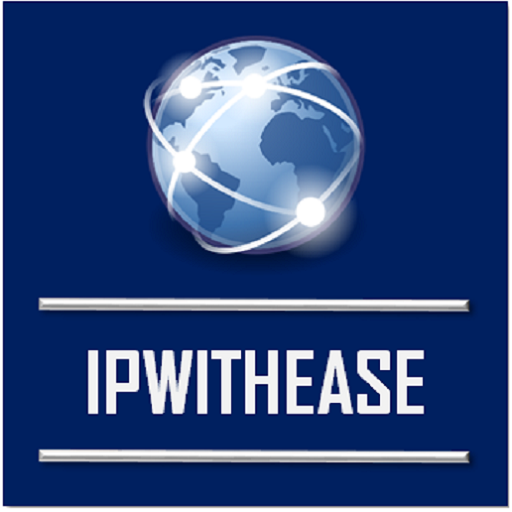
IPwithease is aimed at sharing knowledge across varied domains like Network, Security, Virtualization, Software, Wireless, etc.